Exclusive: How NATIX plans to disrupt autonomous driving with decentralized data
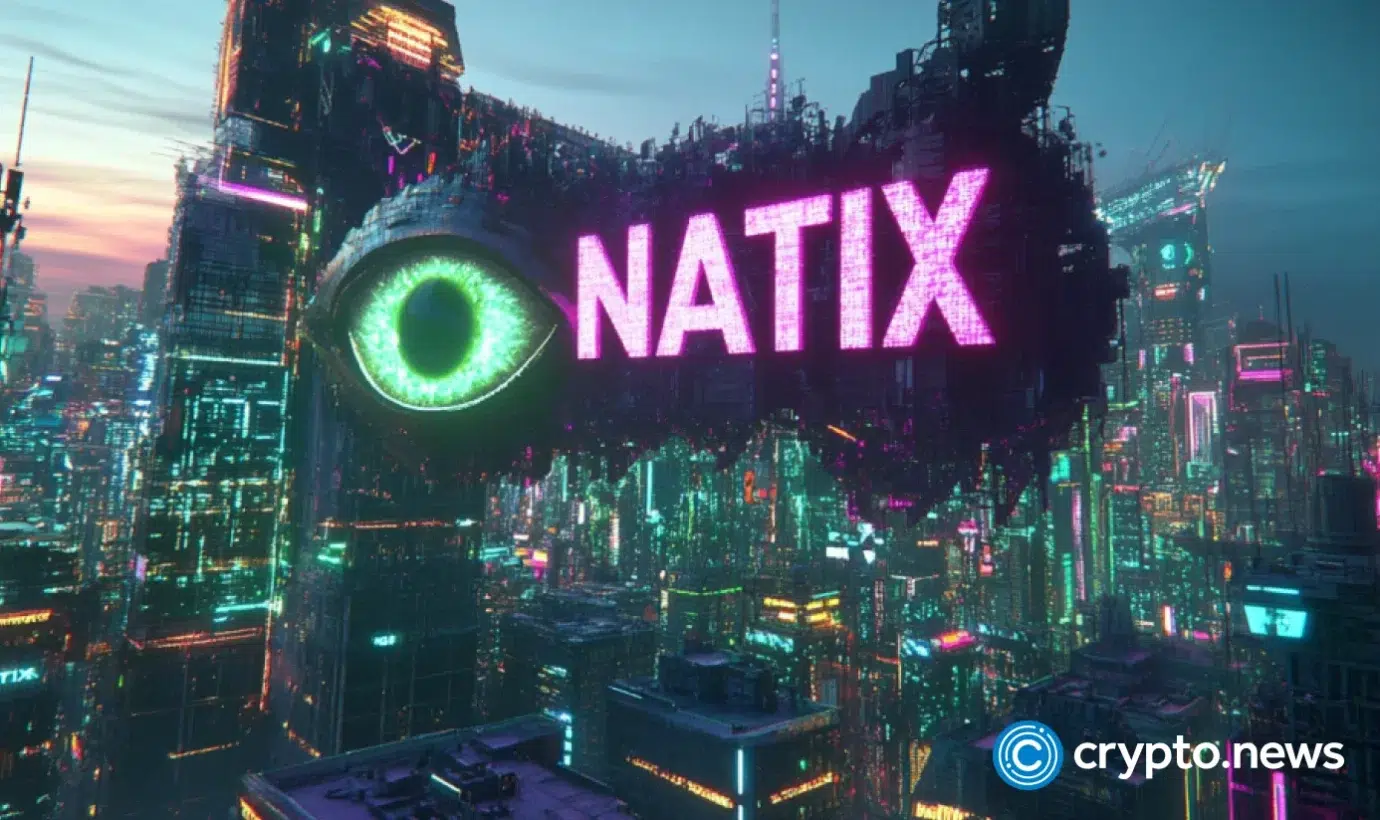
NATIX Network has officially launched StreetVision on the Bittensor decentralized AI network, representing a step forward in its mission to modernize mapping and autonomous driving through decentralized, real-world data processing.
Powered by a Solana- (SOL) based decentralized physical infrastructure network of smart cameras, NATIX combines high-volume, street-level data collection with scalable machine learning through Bittensor (TAO), crypto.news can exclusively report.
The subnet, incubated by Bittensor infrastructure partner Yuma, consumes 360° video feeds captured from NATIX-enabled Tesla vehicles and other mobile devices. It then processes the data into AI models that improve real-time map-making and vehicle autonomy.
The move is consistent with trends seen across the autonomous mobility industry. Notably, companies like Uber are investing in synthetic simulation and bespoke data collections to better manage their self-driving technologies. However, this is expensive and lags behind real-world changes.
To address this, NATIX is eyeing a global community of over 250,000 drivers who collectively logged over 170 million kilometers through smartphone and VX360 device usage.
VX360 and decentralized data training
The VX360, developed in collaboration with Grab, leverages Tesla’s existing camera systems to collect 360° imagery without requiring expensive new hardware. The data is processed both in the cloud and at the edge smartphones detecting elements like traffic lights and signs in real time. More intensive classification is handled off-device.
Through Bittensor’s decentralized framework, NATIX miners are rewarded for training and improving AI models on the subnet, which are then redeployed across the NATIX Edge Network.
“By decentralizing data analysis, we enable the continuous improvement of AI models, significantly enhancing mapping accuracy, autonomous vehicle safety, and real-world responsiveness,” said NATIX CEO and co-founder Alireza Ghods
The subnet’s first focus is roadwork detection, a critical application for both mapping platforms and autonomous vehicle navigation. Over the longer-term, NATIX hopes to expand its operations to include pothole detection and infrastructure analysis. Eventually, NATIX expects to provide a full scenario classification to support autonomous vehicle training.
Q&A with NATIX CEO Alireza Ghods
To better understand the impact of NATIX’s latest launch, crypto.news spoke with Alireza Ghods, CEO and co-founder of NATIX. The conversation focuses on the company’s approach to scaling decentralized data capture, why it believes its data can compete with tech giants, and how it plans to create real demand for its token economy.
The entire Q&A is below:
crypto.news: Tech giants like Waymo, Tesla, and others and even Mobileye have spent years and billions of dollars collecting driving data and developing autonomous tech. What makes NATIX believe it can offer data or AI services at a comparable quality? Crowdsourced data is great for scale, but can crowd-collected camera footage really match the precision of dedicated mapping vehicles?
Alireza Ghods: Data collection for mapping and autonomous driving is super expensive. Large companies that collect this footage are not sharing it as they use that data to have an edge on the product and offering. None of these players have been collecting data at the scale that NATIX can do in a crowdsourced manner. The largest open-source dataset for autonomous driving is Learning to Drive (L2D), a dataset built in collaboration between two projects collected with identical sensor suites installed on 60 EVs operated by driving schools in only German cities over the span of 3 years, which resulted in only 5k hours logged. Since the launch of the VX360 on May 2nd, 2025, we have already collected 2k hours of driving in only 10 days, and with only 1/3 of the total pre-ordered devices live only in the US.
When it comes to the precision in mapping, NATIX may not have the precision and quality of dedicated mapping vehicles, but the sheer size of the network and data collected compensates for it, making the end result as good as data collected by dedicated mapping vehicles. Take Grab, for example. Grab built their whole mapping solution based on crowdsourced efforts, resulting in maps more accurate than Google Maps in SE Asia. For crowdsourced efforts to succeed, you need a large network of drivers/contributors. With over 250K registered drivers, NATIX does have such a network, and it’s the biggest camera DePIN network globally.
CN: Collecting 360° video from potentially thousands (if not more) of cars and phones in real time screams of a massive technical challenge. How is NATIX handling data ingestion and processing at scale?
AG: For data collected with the VX360, the compute we perform for ScenGen is quite heavy and cannot be done at the Edge. We do the processing at the cloud level. The data shared by users is only uploaded when they’re connected to their home Wi-Fi connection.
With that said, we use our smartphone network, which has edge compute power, to detect map attributes such as traffic signs and traffic lights in real-time.
CN: Can you describe the scaling strategy: how will you go from a pilot with a few hundred Teslas or phones to a deployment with, say, 100k+ devices streaming data, without the system breaking or costs skyrocketing?
AG: The VX360 device is quite cost-effective. With a 350$ device, we tap into 360° street-level imagery, while also providing utility for the drivers. Our strategy is tapping into commodity hardware. Everyone owns a smartphone, and Tesla vehicles already come equipped with 360° cameras, so it’s just a matter of tapping into what’s already out there. If we wanted to build a 360° camera, it would be 20-100x more expensive than VX360 in terms of hardware cost, and it would not be scalable as a crowd-sourced solution.
As for further scaling, we are also in discussion with partners who want to operate a fleet of VX360s. This would be fleet owners or integrators who want to sponsor the cost of the device for reward sharing. As an example, we are in discussion with a Tesla fleet owner with over 3,000 Tesla vehicles in their network. We are also in the process of building fleet management functionalities for the fleet operators to increase the utility of our product and go beyond crypto rewards.
CN: Following up from the above question, do you see these big players as customers of NATIX’s data (i.e. you’ll sell them supplemental data) or as competitors you need to outperform? If it’s the latter, where specifically do you think your decentralized approach gives you an edge against far better-funded operations with full control of their fleets?
AG: We have Grab as our customer, and we are in the process of closing a few of the largest autonomous driving players, both as direct customers for the data and partners for building various products for autonomous driving.
Every big player is a potential customer, if not now, then in the future. Some of the bigger players have invested millions into collecting their own datasets, which soon will become outdated as the roads are constantly changing. Even if some tell us they do not need our data today, they will need it soon enough.
Nevertheless, the AD companies that have invested in their own datasets are not larger than 20% of the market. The rest have either not spent much capital to collect data and will need NATIX’s data, or they’re currently relying on their customers’ data (if any), but cannot use it to improve the quality of their models for other customers. This is a real bottleneck for these companies to create a holistic AD stack that can be monetized for all automotive clients.
CN: NATIX isn’t alone in trying to decentralize physical infrastructure. We’ve seen projects like Hivemapper dominate headlines several years back or DIMO (vehicle data) attempt similar crowdsourced models. Their road to success (hehe, pun intended) has been rough with limited user uptake, token value collapse, etc. What lessons has NATIX’s learned or what approach are you taking that differs from those that struggled?
AG: There are also successful models like Geodnet. I believe the winner here is the one that focuses on protocol revenue and has a proper mechanism for value accrual to the token (e.g. buyback and burn). Many of these projects suffer from a lack of Protocol revenue or poor tokenomics (lack of value accrual). In this case, NATIX is closer to Geodnet and is following the same strategy.
Furthermore, you have to look at each business model individually. For example, Hivemapper’s front-facing dashcams collect high-quality street-level imagery for mapping, but NATIX’s 360° data can cater not only to mapping but also to autonomous driving and physical AI. 360° video data is the holy grail of street-level visual data, and not only is it worth 5-10x more than Hivemapper’s data, but it opens the door to countless new mapmaking use cases (e.g., POI detection with side cameras).
Moreover, the 360° data collected can be used for Physical AI, which is a much bigger topic (ever since NVIDIA’s CEO Jensen Huang’s big announcement on the stage at CES 2025). NATIX offers data diversity suitable for the training, testing, and validation of the autonomous driving stack and Physical AI at a fraction of the cost of centralized solutions that companies like Nvidia and Uber invested millions to develop. This data is a painkiller for the simulation-to-reality and reality-to-simulation process in Physical AI development.
That is why we believe we’re solving a much bigger problem, which the industry is ready to pay for TODAY.. Note that cars operate with a surrounding camera system; therefore, training, testing, and validation of the autonomous driving stack is only possible with 360° data and cannot be done with front-facing dashcam data.
CN: Beyond being a reward mechanism, what is the actual utility of the $NATIX token within this ecosystem? Will data purchasers or AI developers need to use $NATIX or TAO tokens to access StreetVision’s services? If not, what will drive demand for $NATIX?
AG: These are two separate ecosystems with different functionality. NATIX is focusing on data curation, while the StreetVision Subnet focuses on (certain) insight extraction and AI model creation.
Each of these ecosystems generates its own value and has its own value accrual mechanism for their token. If anyone is interested in the NATIX data, the value accrual goes towards the $NATIX token through the Protocol Revenue and buyback and burn mechanism. For those interested in the insights or AI models generated by the StreetVision Subnet, the revenue generated will be used for value accrual towards $dTAO (and partially towards $NATIX as the data that was used for such insight and/or models belongs is provided by NATIX).
Additionally, $NATIX is used for the protocol governance and securing the network. This is done via our staking platform, which enables users to participate in protocol governance. Validators in the StreetVision Subnet are also required to stake $NATIX.
CN: Your strategic partnership with Yuma adds a level of credibility, but it also raises centralization concerns. DCG has actually invested in NATIX, and Yuma has been instrumental in launching Bittensor subnets. How do you ensure that neither Yuma nor other heavyweight backers will dominate Subnet 72’s direction or rewards?
AG: Anyone can run a validator for our subnet. Indeed, we are working with two other major Bittensor validator players that will also run validator nodes. Also, we removed staking or $dTAO holding requirements for miners as we wanted to ensure an open ecosystem. As you know, miner centralization is even a bigger concern than validator as the output of the network depends on them.
CN: What current use cases can you point to for the StreetVision Subnet? For example, is Grab already consuming NATIX’s data to update their maps in Southeast Asia right now, or is that just an MOU for future collaboration?
AG: StreetVision’s first task will be real-time roadwork detection. Detecting and classifying roadwork in real-time is crucial for mapping updates and AV reliability. More use cases added later will include detecting potholes, road signs, littering, and infrastructure monitoring. StreetVision Subnet will also later include analysis and classification of driving videos into scenarios from routine traffic conditions to rare “edge cases,” as scenario classification is super important for scenario generation for autonomous driving and Physical AI.
Grab is currently using NATIX’s data to build their pipelines for the US and EU markets, and they are a paying customer already.
As the VX360 just went live on May 2nd, we need some initial data to build sample datasets, which are required by autonomous driving customers. We are in commercial negotiations with dozens of autonomous driving companies. We are also working with a few of the top autonomous driving research labs to create cutting-edge products for simulation-to-reality and reality-to-simulation, which we will announce in the coming months.