Trust, but verify (with better data): overcoming AI’s hallucination problem | Opinion
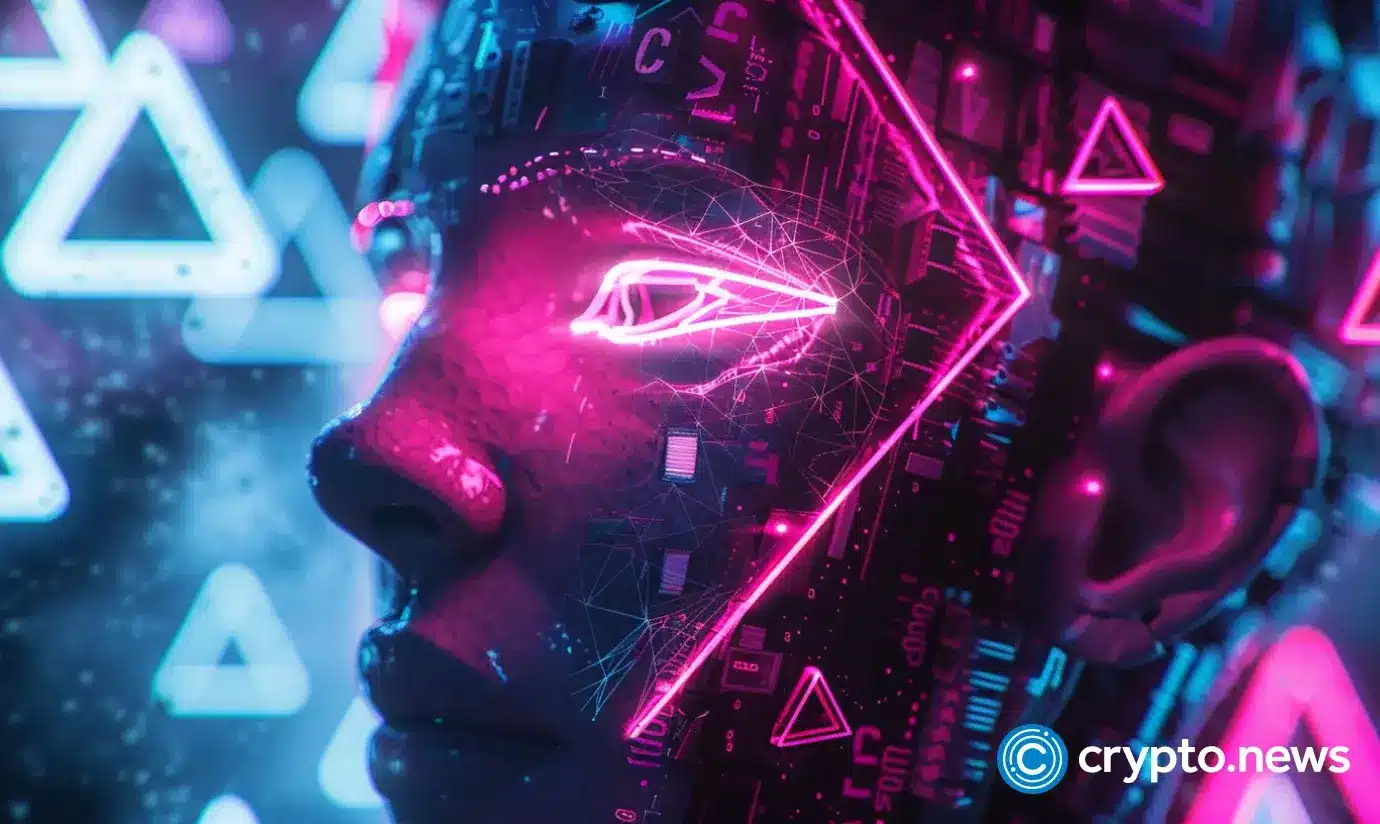
Disclosure: The views and opinions expressed here belong solely to the author and do not represent the views and opinions of crypto.news’ editorial.
The world’s leading online dictionary, Dictionary.com, recently had an interesting choice for its word of the year for 2023: hallucinate. This isn’t due to some panic around a new type of hallucinogen or a new movement of mass hysteria but because of a very peculiar name for a very peculiar phenomenon arising from the emergent industry of artificial intelligence, or more precisely, artificial general intelligence (AGI), which has taken off in the public consciousness since OpenAI’s launch of generative AI chatbot ChatGPT in November 2022.
Of course, only living organisms with actual senses can “hallucinate,” but this is the catch-all term that has been used to describe when an artificial intelligence provides false information or generates random language that does not address the particular query it has been given.
In one case, the artificial intelligence in Microsoft’s Bing search engine began ignoring a New York Times reporter’s queries while attempting to persuade him to leave his wife. Outside of that amusing curiosity (maybe not so much for the reporter), early AGI’s hallucinations have created real problems when users of query engines like ChatGPT unquestioningly accept its responses. In one case, attorneys were fined (and laughed out of the courtroom) for using ChatGPT to construct a legal brief filled with several false case citations.
Those lawyers created short-term financial pain and undoubtedly some long-term personal and professional embarrassment for themselves, but what happens when millions and perhaps even billions are at stake?
AI and financial sector
We have to be careful about the lure of artificial intelligence, especially in a financial industry that has thrived on automation, but has also already suffered significant losses from it as well. If we are going to make this new data analysis tool a part of our information infrastructure moving forward, and especially for our financial information infrastructure moving forward, we have to be wary about how these technologies are implemented and self-regulated within the industry.
Not many people can forget the early—and sometimes perilous—days of automated high-frequency trading, such as when an algorithm wiped out nearly half a billion dollars worth of value from the New York Stock Exchange in 2012. The false data presented by potential AGI hallucinations, wrapped in conversant and human-like language, can be even more risky, not only propagating false data that can exacerbate poorly informed trades and financial panics but also persuade human traders to make other, longer-term errors in judgment.
Why are hallucinations created? Sometimes, the way prompts are constructed can confuse current iterations of generative AI or large language models (LLM). In the same way, smart speakers like Google Home or Amazon Echo can misinterpret background noise as a query that is being directed to them.
More often than not, it is also a case where early AGIs have been trained on a flawed dataset, either through mislabeling or miscategorization. This is more than just a case where different sides of the political aisle have their own definition of “alternative facts” or “fake news” or choose to emphasize the news that makes their side look good and the other side look bad; the AGI simply does not have enough data in its model to provide a direct or coherent answer, so it goes down the rabbit hole of providing an incoherent and indirect one.
In a way, it’s not unlike other nascent technology that came before it, with ambition that outpaced the existing quality and speed of data delivery. The internet did not truly become a game changer until it could transport significant quantities of data from one personal computer to another, and some would argue that the game really changed when our cellular phones could do the same. This new AGI is also training humans to keep building to provide these new AI models with better datasets and more efficient ways of providing fast, usable, and hopefully coherent insights and intelligence.
Is there a solution?
Many have suggested different ways to minimize hallucinations, including something called a retrieval-augmented generation (RAG), which is essentially a way of continually updating data sources in real time. This could be one advantage of Elon Musk’s Grok AI, which has access to the most popular public real-time data source of the last 15 years.
I’m partial to blockchain as a solution, though. It wouldn’t be locked into one corporate gatekeeper or walled data garden and can build up new and better sources of decentralized data. Blockchain is built not just for peer-to-peer data storage and transmission but also for payment transmission, which could create new methods of incentivization for what is certain to be a radical new stage of an AI-infused information economy.
In the world of finance, something like a decentralized knowledge graph would empower and incentivize stakeholders across the industry to share more data transparently. Blockchain would be able to update and verify all relevant and immutable information in real-time. This data verification method would be a supercharged version of RAG and drastically reduce the number of AGI hallucinations, with knowledge assets having embedded semantics and verifiability (In the interest of disclosure, I have worked with OriginTrail, which is developing its version of a decentralized knowledge graph).
There might be one day when “robots” are even better traders than people. Ultimately, it will be our choice whether to create a system that provides those robots with the tools to be better, more robust, and faster in the reality we create and not the one they are “hallucinating.”